Big Data UX
Establish behavior patterns that allow feeding recommendation systems or adapting the software to a target audience
Project co-financed by the European Regional Development Fund (ERDF) and the Society for Regional Development of Cantabria (SODERCAN) within the program “I+C=+C 2016 – R&D PROJECTS IN THE FIELD OF ICT, SMART LINE ”, with reference identifier TI16-IN-007.l 50%.
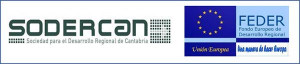
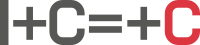
Introduction
Ubiquitous computing environments are those in which interaction with technology occurs at any time and place, sometimes in an invisible way for the user, through any type of device. Multiple technological advances favor the appearance of these environments, such as those related to home automation, wearables or smart cities, in addition to the enormous growth in the use of portable devices such as smartphones or tablets.
All this interaction produces large volumes of data that can be exploited to extract and understand user habits and behaviors. And the acquisition of this knowledge can allow the technology to evolve according to the needs of the user.
Context
Among the many possible ubiquitous contexts, the broadest and most entrenched is the one that arises from interaction through smartphones and mobile applications (or apps). These devices, already in daily use, collect a quantity of data of a varied nature, from the use of user interfaces to geolocation histories or NFC (Near Field Communication) interaction.
The registered information has a versatile richness, from which a large number of usability patterns, mobility and preferences can be extracted, and with the potential to inform about the characteristics of a community of users, feed recommendation systems or adapt the software to an audience. objective. However, special treatment is required for effective knowledge discovery given the high dimensions and constant growth of data.
Project Objectives
The objective of this project is to take advantage of the user patterns that appear in these mobile environments. To do this, the use of machine learning and learning by observation (Learning From Observation) techniques is proposed for the creation of behavioral models from the history of interactions.
The models, with predictive and generative capabilities, will collect the user’s patterns and particularities and will subsequently allow, through unsupervised learning, to segment a community of users into different profiles with a series of typical behaviors.
With the techniques developed, it is desired to obtain a software system that generates specifications in natural language about the user profiles of an app. The generated descriptions will provide a competitive advantage to adjust the services and features of an app to its audience or its different sectors, thus seeking a substantial improvement in the user experience. Within this framework, the user, through their normal activity in an app, can have a much more significant role in its progress.
Proyecto cofinanciado por el Fondo Europeo de Desarrollo Regional (FEDER) y la Sociedad para el Desarrollo Regional de Cantabria (SODERCAN) dentro del programa “I+C=+C 2016 – PROYECTOS DE I+D EN EL ÁMBITO DE LAS TIC, LÍNEA SMART”, con identificador de referencia TI16-IN-007.
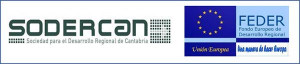
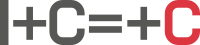
Introducción
Los entornos de computación ubicua son aquellos en los que la interacción con la tecnología se produce en cualquier momento y lugar, en ocasiones de forma invisible para el usuario, a través de cualquier tipo de dispositivo. Múltiples avances tecnológicos propician la aparición de estos entornos, como los relativos a domótica, wearables o smart cities, además del enorme crecimiento del empleo de dispositivos portátiles como teléfonos inteligentes o tablets.
Toda esta interacción produce grandes volúmenes de datos que pueden ser explotados para extraer y entender hábitos y conductas de los usuarios. Y la adquisición de este conocimiento puede permitir a la tecnología evolucionar según las necesidades del propio usuario.
Contexto
De entre los numerosos contextos ubicuos posibles, el más amplio y arraigado es el que surge de la interacción a través de la telefonía inteligente y aplicaciones móviles (o apps). Estos dispositivos, ya de un uso cotidiano, recogen una cantidad de datos de carácter variado, desde el uso de las interfaces de usuario a historiales de geolocalización o interacción NFC (Near Field Communication).
La información registrada tiene una riqueza versátil, de donde se puede extraer gran cantidad de patrones de usabilidad, movilidad y preferencias, y con el potencial de informar sobre las características de una comunidad de usuarios, alimentar sistemas de recomendación o adaptar el software a un público objetivo. Sin embargo, se requiere un tratamiento especial para el descubrimiento de conocimiento efectivo dadas las elevadas dimensiones y crecimiento constante de los datos.
Objetivos del proyecto
El objetivo de este proyecto es el aprovechamiento de los patrones de usuario que aparecen en estos entornos móviles. Para ello, se plantea la utilización de técnicas de aprendizaje automático y aprendizaje por observación (Learning From Observation) para el creación de modelos del comportamiento a partir del historial de interacciones.
Los modelos, con capacidades predictivas y generativas, recogerán los patrones y particularidades del usuario y permitirán posteriormente mediante aprendizaje no-supervisado segmentar una comunidad de usuarios en diferentes perfiles con una serie de comportamientos típicos.
Con las técnicas desarrolladas, se desea obtener un sistema software que genere especificaciones en lenguaje natural sobre los perfiles de usuario de una app. Las descripciones generadas proporcionarán una ventaja competitiva para ajustar los servicios y características de una app a su público o los distintos sectores del mismo, buscando así una mejora sustancial en la experiencia del usuario. Dentro de este marco, el usuario a través de su actividad normal en una app puede tener un papel mucho más significativo en el progreso de la misma.