Bayesian SDN
Application of Bayesian diagnostic techniques in SDN networks (Software-Defined Networks)
Project co-financed by the Ministry of Energy, Tourism and Digital Agenda (MINETAD) and by the European Regional Development Fund (ERDF), within the framework of the 2016 call for the Strategic Action for Digital Economy and Society, reference number of the TSI project -100102-2016-12
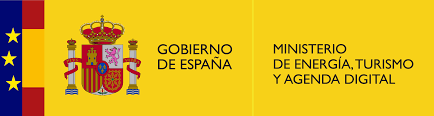
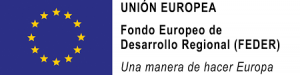
Technological background
Network management is one of the most expensive tasks for telecommunications operating companies. Consequently, there is a tendency to delegate network management to the network itself. This is known as Autonomic Networking. But today, fault diagnosis is still a non-autonomous task. Traditionally, this process has been carried out by human experts supported by monitoring systems to detect alarms or symptoms. But even with those systems, the diagnostic task is essentially a manual process.
The constant increase in network size and complexity makes fault diagnosis a critical business task that must be managed quickly and reliably. To carry it out, highly-skilled engineers are required, but even these people are not always able to cope with the growing heterogeneity and complexity of networks, since diagnosis is a difficult process, time-consuming and, therefore, it is an expensive task. Consequently, operators are aiming to fully automate fault diagnosis to reduce the cost of operation and improve the customer experience through the automated operation of standardized diagnostic processes.
Project Objectives
In order to solve the aforementioned limitations and advance in the development of automatic diagnostic techniques for SDN networks, this project aims to generate different monitoring and diagnostic techniques based on artificial intelligence techniques that allow the system to learn or adapt to the changes and evolution of the network. To do this, they plan to study and evaluate different evolutionary computing techniques or machine learning techniques. On the one hand, evolutionary computing techniques are very attractive and used in the literature to solve different optimization problems, which is interesting to evaluate and/or monitor the state of the network and thus be able to detect different anomalies in real time. On the other hand, machine learning techniques offer the system the ability to learn from the environment with previously collected data, which allows the system’s behavior to be adapted if the environment is modified by external factors.
The development of this project will allow AXPE Consulting to enhance its position as a reference provider in the Telecommunications Sector, while helping us to consolidate our R&D area, as a lever for corporate development and a differentiating element. against our competition.
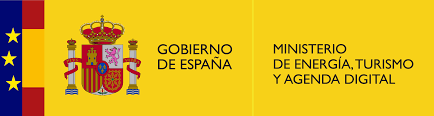
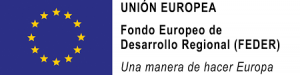
Trasfondo tecnológico
La gestión de la red es una de las tareas más costosas para las empresas operadoras de telecomunicaciones. En consecuencia, hay una tendencia de delegar la gestión de la red en la propia red. Esto se conoce como Autonomic Networking. Pero, hoy en día, el diagnóstico de fallos sigue siendo una tarea no-autónoma. Tradicionalmente, este proceso se ha llevado a cabo por expertos humanos apoyados por sistemas de monitorización para la detección de alarmas o síntomas. Pero, incluso con esos sistemas, la tarea de diagnóstico es fundamentalmente un proceso manual.
El aumento constante en el tamaño y la complejidad de la red hace que el diagnóstico de fallos sea una tarea fundamental para el negocio que debe ser gestionada de manera rápida y fiable. Para llevarla a cabo, se requieren ingenieros altamente cualificados, pero incluso estas personas no siempre son capaces de hacer frente a la creciente heterogeneidad y complejidad de las redes, ya que el diagnóstico es un proceso difícil, requiere mucho tiempo y, por lo tanto, es una tarea costosa. En consecuencia, los operadores tienen el objetivo de automatizar totalmente el diagnóstico de fallos para reducir el coste de operación y mejorar la experiencia de los clientes a través de la operación automatizada de los procesos de diagnóstico estandarizados.
Objetivos del proyecto
Con el fin de solventar las limitaciones mencionadas anteriormente y avanzar en el desarrollo de técnicas de diagnóstico automático para redes SDN, este proyecto tiene como objetivo generar diferentes técnicas de monitorización y diagnóstico basadas en técnicas de inteligencia artificial que permitan al sistema aprender o adaptarse a los cambios y a la evolución de la red. Para ello se plantean estudiar y evaluar diferentes técnicas de computación evolutiva o técnicas de aprendizaje automático. Por un lado, las técnicas de computación evolutiva son técnicas muy atractivas y utilizadas en la literatura para resolver diferentes problemas de optimización, lo cual resulta interesante para evaluar y/o monitorizar el estado de la red y así poder detectar diferentes anomalías en tiempo real. Por otro lado, las técnicas de aprendizaje automático ofrecen la capacidad al sistema de aprender del entorno con datos recolectados con anterioridad, lo cual permite adaptar el comportamiento del sistema si el entorno se ve modificado por factores externos.
El desarrollo de este proyecto va a permitir a AXPE Consulting potenciar su posicionamiento como proveedor de referencia en el Sector de la Telecomunicaciones, al mismo tiempo que nos ayuda a consolidar nuestra área de I+D+i, como palanca de desarrollo corporativo y elemento diferenciador frente a nuestra competencia.